생성 AI란 무엇이며 어떻게 작동하나요?
게시 됨: 2023-09-26인공지능(AI)의 하위 집합인 제너레이티브 AI(Generative AI)가 기술 세계에서 혁명적인 힘으로 떠올랐습니다. 하지만 정확히 무엇입니까? 그리고 왜 그렇게 많은 주목을 받는 걸까요?
이 심층 가이드에서는 생성 AI 모델의 작동 방식, 수행할 수 있는 작업과 수행할 수 없는 작업, 이러한 모든 요소의 의미에 대해 자세히 알아봅니다.
생성 AI란 무엇인가?
제너레이티브 AI(genAI)는 텍스트, 이미지, 음악, 비디오 등 새로운 콘텐츠를 생성할 수 있는 시스템을 의미합니다. 전통적으로 AI/ML은 지도 학습, 비지도 학습, 강화 학습이라는 세 가지를 의미했습니다. 각각은 클러스터링 결과를 기반으로 통찰력을 제공합니다.
비생성 AI 모델은 입력을 기반으로 계산을 수행합니다(예: 이미지 분류 또는 문장 번역). 이와 대조적으로 생성 모델은 에세이 작성, 음악 작곡, 그래픽 디자인, 심지어 현실 세계에 존재하지 않는 사실적인 인간 얼굴 생성과 같은 "새로운" 출력을 생성합니다.
생성 AI의 의미
생성적 AI의 부상은 중요한 의미를 갖습니다. 콘텐츠 생성 능력을 통해 엔터테인먼트, 디자인, 저널리즘과 같은 산업은 패러다임 변화를 목격하고 있습니다.
예를 들어, 통신사는 AI를 사용하여 보고서 초안을 작성할 수 있고, 디자이너는 그래픽에 대한 AI 지원 제안을 받을 수 있습니다. AI는 해당 옵션이 좋은지 여부에 관계없이 몇 초 안에 수백 개의 광고 슬로건을 생성할 수 있습니다. 아니면 다른 문제입니다.
제너레이티브 AI는 개별 사용자를 위한 맞춤형 콘텐츠를 제작할 수 있습니다. 기분에 따라 독특한 노래를 작곡하는 음악 앱이나 관심 있는 주제에 대한 기사 초안을 작성하는 뉴스 앱 등을 생각해 보세요.
문제는 AI가 콘텐츠 제작에 더욱 필수적인 역할을 하게 되면서 진정성, 저작권, 인간 창의성의 가치에 대한 의문이 더욱 널리 퍼지고 있다는 점이다.
생성 AI는 어떻게 작동하나요?
Generative AI의 핵심은 그것이 문장의 다음 단어이든 이미지의 다음 픽셀이든 상관없이 시퀀스의 다음 데이터 조각을 예측하는 것입니다. 이것이 어떻게 달성되는지 분석해 보겠습니다.
통계 모델
통계 모델은 대부분의 AI 시스템의 중추입니다. 그들은 다양한 변수 사이의 관계를 표현하기 위해 수학 방정식을 사용합니다.
생성적 AI의 경우 모델은 데이터의 패턴을 인식한 다음 이러한 패턴을 사용하여 생성하도록 훈련됩니다. 새롭고 유사한 데이터.
모델이 영어 문장에 대해 학습되면 한 단어가 다른 단어 뒤에 올 확률의 통계적 가능성을 학습하여 일관된 문장을 생성할 수 있습니다.
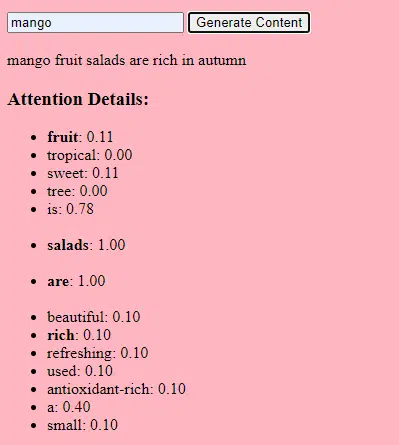
데이터 수집
데이터의 질과 양 모두 중요합니다. 생성 모델은 패턴을 이해하기 위해 방대한 데이터 세트에서 훈련됩니다.
언어 모델의 경우 이는 책, 웹 사이트 및 기타 텍스트에서 수십억 개의 단어를 섭취하는 것을 의미할 수 있습니다.
이미지 모델의 경우 수백만 개의 이미지를 분석한다는 의미일 수 있습니다. 훈련 데이터가 더 다양하고 포괄적일수록 모델은 다양한 출력을 더 잘 생성합니다.
변환기와 주의가 작동하는 방식
Transformers는 Vaswani 등의 2017년 "Attention Is All You Need"라는 제목의 논문에 소개된 일종의 신경망 아키텍처입니다. 이후 그들은 대부분의 최첨단 언어 모델의 기초가 되었습니다. ChatGPT는 변환기 없이는 작동하지 않습니다.
"주의" 메커니즘을 사용하면 인간이 문장을 이해할 때 특정 단어에 주의를 기울이는 것과 마찬가지로 모델이 입력 데이터의 다양한 부분에 집중할 수 있습니다.
이 메커니즘을 통해 모델은 입력의 어느 부분이 특정 작업과 관련이 있는지 결정할 수 있으므로 매우 유연하고 강력해집니다.
아래 코드는 변환기 메커니즘의 기본 분석으로, 각 부분을 일반 영어로 설명합니다.
class Transformer: # Convert words to vectors # What this is : turns words into "vector embeddings" –basically numbers that represent the words and their relationships to each other. # Demo : "the pineapple is cool and tasty" -> [0.2, 0.5, 0.3, 0.8, 0.1, 0.9] self.embedding = Embedding(vocab_size, d_model) # Add position information to the vectors # What this is : Since words in a sentence have a specific order, we add information about each word's position in the sentence. # Demo : "the pineapple is cool and tasty" with position -> [0.2+0.01, 0.5+0.02, 0.3+0.03, 0.8+0.04, 0.1+0.05, 0.9+0.06] self.positional_encoding = PositionalEncoding(d_model) # Stack of transformer layers # What this is : Multiple layers of the Transformer model stacked on top of each other to process data in depth. # Why it does it : Each layer captures different patterns and relationships in the data. # Explained like I'm five : Imagine a multi-story building. Each floor (or layer) has people (or mechanisms) doing specific jobs. The more floors, the more jobs get done! self.transformer_layers = [TransformerLayer(d_model, nhead) for _ in range(num_layers)] # Convert the output vectors to word probabilities # What this is : A way to predict the next word in a sequence. # Why it does it : After processing the input, we want to guess what word comes next. # Explained like I'm five : After listening to a story, this tries to guess what happens next. self.output_layer = Linear(d_model, vocab_size) def forward(self, x): # Convert words to vectors, as above x = self.embedding(x) # Add position information, as above x = self.positional_encoding(x) # Pass through each transformer layer # What this is : Sending our data through each floor of our multi-story building. # Why it does it : To deeply process and understand the data. # Explained like I'm five : It's like passing a note in class. Each person (or layer) adds something to the note before passing it on, which can end up with a coherent story – or a mess. for layer in self.transformer_layers: x = layer(x) # Get the output word probabilities # What this is : Our best guess for the next word in the sequence. return self.output_layer(x)
코드에는 Transformer 클래스와 단일 TransformerLayer 클래스가 있을 수 있습니다. 이는 건물 전체와 바닥에 대한 청사진을 갖는 것과 같습니다.
이 TransformerLayer 코드 조각은 다중 헤드 주의 및 특정 배열과 같은 특정 구성 요소가 작동하는 방식을 보여줍니다.
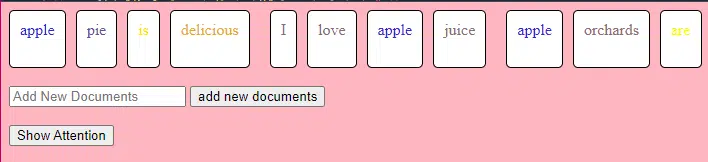
class TransformerLayer: # Multi-head attention mechanism # What this is : A mechanism that lets the model focus on different parts of the input data simultaneously. # Demo : "the pineapple is cool and tasty" might become "this PINEAPPLE is COOL and TASTY" as the model pays more attention to certain words. self.attention = MultiHeadAttention(d_model, nhead) # Simple feed-forward neural network # What this is : A basic neural network that processes the data after the attention mechanism. # Demo : "this PINEAPPLE is COOL and TASTY" -> [0.25, 0.55, 0.35, 0.85, 0.15, 0.95] (slight changes in numbers after processing) self.feed_forward = FeedForward(d_model) def forward(self, x): # Apply attention mechanism # What this is : The step where we focus on different parts of the sentence. # Explained like I'm five : It's like highlighting important parts of a book. attention_output = self.attention(x, x, x) # Pass the output through the feed-forward network # What this is : The step where we process the highlighted information. return self.feed_forward(attention_output)
피드포워드 신경망은 가장 간단한 유형의 인공 신경망 중 하나입니다. 입력층, 하나 이상의 은닉층, 출력층으로 구성됩니다.
데이터는 입력 레이어에서 히든 레이어를 거쳐 출력 레이어로 한 방향으로 흐릅니다. 네트워크에는 루프나 사이클이 없습니다.
변환기 아키텍처의 맥락에서 피드포워드 신경망은 각 계층의 주의 메커니즘 이후에 사용됩니다. 이는 ReLU 활성화를 사이에 두고 간단한 2계층 선형 변환입니다.
# Scaled dot-product attention mechanism class ScaledDotProductAttention: def __init__(self, d_model): # Scaling factor helps in stabilizing the gradients # it reduces the variance of the dot product. # What this is: A scaling factor based on the size of our model's embeddings. # What it does : Helps to make sure the dot products don't get too big. # Why it does it : Big dot products can make a model unstable and harder to train. # How it does it : By dividing the dot products by the square root of the embedding size. # It's used when calculating attention scores. # Explained like I'm five : Imagine you shouted something really loud. This scaling factor is like turning the volume down so it's not too loud. self.scaling_factor = d_model ** 0.5 def forward(self, query, key, value): # What this is : The function that calculates how much attention each word should get. # What it does : Determines how relevant each word in a sentence is to every other word. # Why it does it : So we can focus more on important words when trying to understand a sentence. # How it does it : By taking the dot product (the numeric product: a way to measure similarity) of the query and key, then scaling it, and finally using that to weigh our values. # How it fits into the rest of the code : This function is called whenever we want to calculate attention in our model. # Explained like I'm five : Imagine you have a toy and you want to see which of your friends likes it the most. This function is like asking each friend how much they like the toy, and then deciding who gets to play with it based on their answers. # Calculate attention scores by taking the dot product of the query and key. scores = dot_product(query, key) / self.scaling_factor # Convert the raw scores to probabilities using the softmax function. attention_weights = softmax(scores) # Weight the values using the attention probabilities. return dot_product(attention_weights, value) # Feed-forward neural network # This is an extremely basic example of a neural network. class FeedForward: def __init__(self, d_model): # First linear layer increases the dimensionality of the data. self.layer1 = Linear(d_model, d_model * 4) # Second linear layer brings the dimensionality back to d_model. self.layer2 = Linear(d_model * 4, d_model) def forward(self, x): # Pass the input through the first layer, #Pass the input through the first layer: # Input : This refers to the data you feed into the neural network. I # First layer : Neural networks consist of layers, and each layer has neurons. When we say "pass the input through the first layer," we mean that the input data is being processed by the neurons in this layer. Each neuron takes the input, multiplies it by its weights (which are learned during training), and produces an output. # apply ReLU activation to introduce non-linearity, # and then pass through the second layer. #ReLU activation: ReLU stands for Rectified Linear Unit. # It's a type of activation function, which is a mathematical function applied to the output of each neuron. In simpler terms, if the input is positive, it returns the input value; if the input is negative or zero, it returns zero. # Neural networks can model complex relationships in data by introducing non-linearities. # Without non-linear activation functions, no matter how many layers you stack in a neural network, it would behave just like a single-layer perceptron because summing these layers would give you another linear model. # Non-linearities allow the network to capture complex patterns and make better predictions. return self.layer2(relu(self.layer1(x))) # Positional encoding adds information about the position of each word in the sequence. class PositionalEncoding: def __init__(self, d_model): # What this is : A setup to add information about where each word is in a sentence. # What it does : Prepares to add a unique "position" value to each word. # Why it does it : Words in a sentence have an order, and this helps the model remember that order. # How it does it : By creating a special pattern of numbers for each position in a sentence. # How it fits into the rest of the code : Before processing words, we add their position info. # Explained like I'm five : Imagine you're in a line with your friends. This gives everyone a number to remember their place in line. pass def forward(self, x): # What this is : The main function that adds position info to our words. # What it does : Combines the word's original value with its position value. # Why it does it : So the model knows the order of words in a sentence. # How it does it : By adding the position values we prepared earlier to the word values. # How it fits into the rest of the code : This function is called whenever we want to add position info to our words. # Explained like I'm five : It's like giving each of your toys a tag that says if it's the 1st, 2nd, 3rd toy, and so on. return x # Helper functions def dot_product(a, b): # Calculate the dot product of two matrices. # What this is : A mathematical operation to see how similar two lists of numbers are. # What it does : Multiplies matching items in the lists and then adds them up. # Why it does it : To measure similarity or relevance between two sets of data. # How it does it : By multiplying and summing up. # How it fits into the rest of the code : Used in attention to see how relevant words are to each other. # Explained like I'm five : Imagine you and your friend have bags of candies. You both pour them out and match each candy type. Then, you count how many matching pairs you have. return a @ b.transpose(-2, -1) def softmax(x): # Convert raw scores to probabilities ensuring they sum up to 1. # What this is : A way to turn any list of numbers into probabilities. # What it does : Makes the numbers between 0 and 1 and ensures they all add up to 1. # Why it does it : So we can understand the numbers as chances or probabilities. # How it does it : By using exponentiation and division. # How it fits into the rest of the code : Used to convert attention scores into probabilities. # Explained like I'm five : Lets go back to our toys. This makes sure that when you share them, everyone gets a fair share, and no toy is left behind. return exp(x) / sum(exp(x), axis=-1) def relu(x): # Activation function that introduces non-linearity. It sets negative values to 0. # What this is : A simple rule for numbers. # What it does : If a number is negative, it changes it to zero. Otherwise, it leaves it as it is. # Why it does it : To introduce some simplicity and non-linearity in our model's calculations. # How it does it : By checking each number and setting it to zero if it's negative. # How it fits into the rest of the code : Used in neural networks to make them more powerful and flexible. # Explained like I'm five : Imagine you have some stickers, some are shiny (positive numbers) and some are dull (negative numbers). This rule says to replace all dull stickers with blank ones. return max(0, x)
생성 AI의 작동 방식 – 간단한 용어로
생성 AI를 가중치 주사위를 굴리는 것으로 생각해보세요. 훈련 데이터는 가중치(또는 확률)를 결정합니다.
주사위가 문장의 다음 단어를 나타내는 경우 훈련 데이터에서 현재 단어 뒤에 자주 오는 단어의 가중치가 더 높습니다. 따라서 "하늘"은 "바나나"보다 "파랑" 뒤에 더 자주 올 수 있습니다. AI가 콘텐츠를 생성하기 위해 "주사위를 굴릴" 때 훈련을 기반으로 통계적으로 더 가능성 있는 시퀀스를 선택할 가능성이 더 높습니다.
그렇다면 LLM은 어떻게 독창적인 것처럼 보이는 콘텐츠를 생성할 수 있습니까?
"콘텐츠 마케팅 담당자를 위한 최고의 Eid al-Fitr 선물"인 가짜 목록을 가지고 LLM이 어떻게 생성할 수 있는지 살펴보겠습니다. 선물, Eid 및 콘텐츠 마케팅 담당자에 대한 문서의 텍스트 단서를 결합하여 이 목록을 만듭니다.
처리하기 전에 텍스트는 '토큰'이라는 작은 조각으로 나뉩니다. 이러한 토큰은 한 문자만큼 짧을 수도 있고 한 단어만큼 길 수도 있습니다.
예: “Eid al-Fitr는 축하입니다”는 [“Eid”, “al-Fitr”, “is”, “a”, “celebration”]이 됩니다.
이를 통해 모델은 관리 가능한 텍스트 덩어리로 작업하고 문장 구조를 이해할 수 있습니다.
그런 다음 각 토큰은 임베딩을 사용하여 벡터(숫자 목록)로 변환됩니다. 이러한 벡터는 각 단어의 의미와 맥락을 포착합니다.
위치 인코딩은 각 단어 벡터에 문장 내 위치에 대한 정보를 추가하여 모델이 이 순서 정보를 잃지 않도록 합니다.
그런 다음 주의 메커니즘을 사용합니다. 이를 통해 모델은 출력을 생성할 때 입력 텍스트의 다른 부분에 집중할 수 있습니다. BERT를 기억하신다면 이것이 Google 직원들에게 BERT에 대해 매우 흥미로웠던 점입니다.
우리 모델이 " 선물 "에 대한 텍스트를 보고 사람들이 축하 행사 중에 선물을 준다는 것을 알고 있으며 " Eid al-Fitr "이 중요한 축하 행사 라는 텍스트도 본 경우 이러한 연결에 " 주의 "를 기울일 것입니다.
마찬가지로, 특정 도구 나 리소스가 필요한 " 콘텐츠 마케팅 담당자 "에 대한 텍스트를 본 경우 " 선물 "이라는 개념을 "콘텐츠 마케팅 담당자 "에 연결할 수 있습니다.
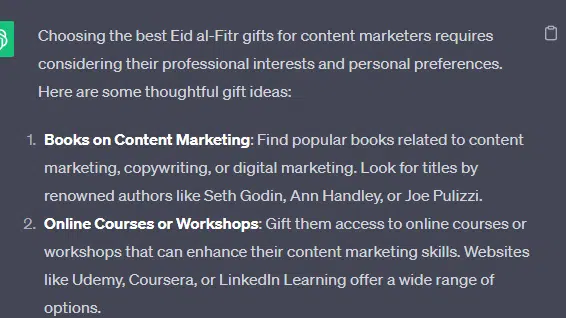
이제 컨텍스트를 결합할 수 있습니다. 모델이 여러 Transformer 레이어를 통해 입력 텍스트를 처리할 때 학습한 컨텍스트를 결합합니다.
따라서 원문에 '콘텐츠 마케터를 위한 이드 알피트르 선물'이 언급되지 않았더라도 모델은 '이드 알피트르', '선물', '콘텐츠 마케터' 개념을 통합하여 이러한 콘텐츠를 생성할 수 있습니다.
이는 각 용어에 대한 더 넓은 맥락을 학습했기 때문입니다.
주의 메커니즘과 각 Transformer 레이어의 피드포워드 네트워크를 통해 입력을 처리한 후 모델은 시퀀스의 다음 단어에 대한 어휘에 대한 확률 분포를 생성합니다.
'최고', '이드 알피트르' 같은 단어 다음에는 '선물'이라는 단어가 올 확률이 높다고 생각할 수도 있습니다. 마찬가지로, "선물"을 "콘텐츠 마케팅 담당자"와 같은 잠재적인 수신자와 연결할 수도 있습니다.
검색 마케팅 담당자가 신뢰하는 일일 뉴스레터를 받아보세요.
약관을 참조하세요.
얼마나 큰 언어 모델이 구축되는지
기본 변환기 모델에서 GPT-3 또는 BERT와 같은 정교한 LLM(대형 언어 모델)으로의 여정에는 다양한 구성 요소를 확장하고 개선하는 작업이 포함됩니다.
단계별 분석은 다음과 같습니다.
LLM은 방대한 양의 텍스트 데이터에 대해 교육을 받았습니다. 이 데이터가 얼마나 방대한지 설명하기는 어렵습니다.
많은 LLM의 출발점인 C4 데이터 세트는 750GB의 텍스트 데이터입니다. 이는 805,306,368,000바이트로 많은 정보입니다. 이 데이터에는 책, 기사, 웹사이트, 포럼, 댓글 섹션 및 기타 소스가 포함될 수 있습니다.
데이터가 다양하고 포괄적일수록 모델의 이해 및 일반화 기능이 향상됩니다.
기본 변환기 아키텍처는 기초로 남아 있지만 LLM에는 훨씬 더 많은 수의 매개변수가 있습니다. 예를 들어 GPT-3에는 1,750억 개의 매개변수가 있습니다. 이 경우 매개변수는 훈련 과정에서 학습되는 신경망의 가중치와 편향을 나타냅니다.
딥 러닝에서 모델은 예측과 실제 결과 간의 차이를 줄이기 위해 이러한 매개변수를 조정하여 예측하도록 훈련됩니다.
이러한 매개변수를 조정하는 프로세스를 최적화라고 하며 경사하강법과 같은 알고리즘을 사용합니다.
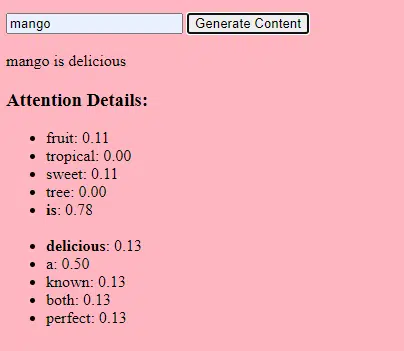
- 가중치: 이는 네트워크 레이어 내의 입력 데이터를 변환하는 신경망의 값입니다. 모델의 출력을 최적화하기 위해 훈련 중에 조정됩니다. 인접한 레이어의 뉴런 사이의 각 연결에는 연관된 가중치가 있습니다.
- 편향: 이는 레이어 변환의 출력에 추가되는 신경망의 값이기도 합니다. 이는 모델에 추가적인 자유도를 제공하여 훈련 데이터에 더 잘 맞도록 합니다. 레이어의 각 뉴런에는 연관된 편향이 있습니다.
이러한 확장을 통해 모델은 데이터에서 더욱 복잡한 패턴과 관계를 저장하고 처리할 수 있습니다.
매개변수 수가 많다는 것은 모델이 훈련과 추론을 위해 상당한 계산 능력과 메모리를 필요로 한다는 것을 의미합니다. 이것이 바로 이러한 모델을 훈련하는 데 리소스 집약적이며 일반적으로 GPU 또는 TPU와 같은 특수 하드웨어를 사용하는 이유입니다.
이 모델은 강력한 계산 리소스를 사용하여 시퀀스의 다음 단어를 예측하도록 훈련되었습니다. 발생한 오류를 기반으로 내부 매개변수를 조정하여 지속적으로 예측을 개선합니다.
우리가 논의한 것과 같은 주의 메커니즘은 LLM의 핵심입니다. 이를 통해 모델은 출력을 생성할 때 입력의 다양한 부분에 집중할 수 있습니다.
어텐션 메커니즘은 맥락에서 다양한 단어의 중요성을 평가함으로써 모델이 일관되고 맥락에 맞는 텍스트를 생성할 수 있도록 해줍니다. 이렇게 대규모로 작업을 수행하면 LLM이 기존 방식대로 작업할 수 있습니다.
변환기는 어떻게 텍스트를 예측합니까?
Transformer는 각 레이어에 주의 메커니즘과 피드포워드 네트워크가 장착된 여러 레이어를 통해 입력 토큰을 처리하여 텍스트를 예측합니다.
처리 후 모델은 시퀀스의 다음 단어에 대한 어휘에 대한 확률 분포를 생성합니다. 일반적으로 확률이 가장 높은 단어가 예측으로 선택됩니다.
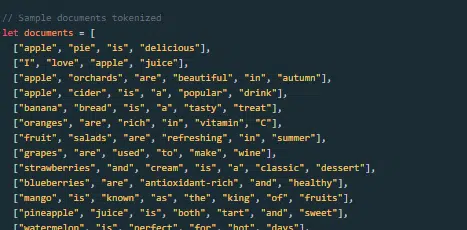
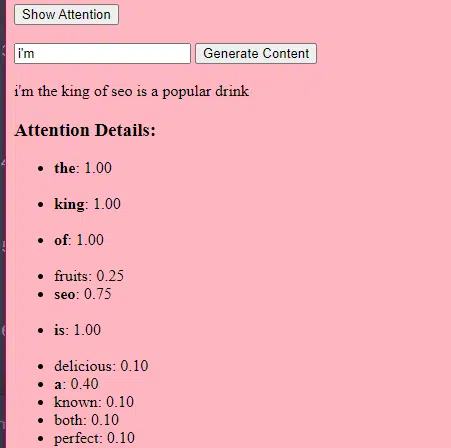
대규모 언어 모델은 어떻게 구축되고 훈련되나요?
LLM 구축에는 데이터 수집, 정리, 모델 교육, 모델 미세 조정 및 강력하고 지속적인 테스트가 포함됩니다.
모델은 처음에 시퀀스의 다음 단어를 예측하기 위해 방대한 코퍼스에 대해 훈련되었습니다. 이 단계를 통해 모델은 문법의 패턴을 선택하는 단어, 세상에 대한 사실을 나타낼 수 있는 관계, 논리적 추론처럼 느껴지는 연결 사이의 연결을 학습할 수 있습니다. 또한 이러한 연결을 통해 훈련 데이터에 존재하는 편향을 포착할 수 있습니다.
사전 학습 후 모델은 더 좁은 데이터 세트에서 개선되며, 종종 인간 검토자가 지침을 따릅니다.
미세 조정은 LLM 구축에서 중요한 단계입니다. 여기에는 보다 구체적인 데이터 세트 또는 작업에 대해 사전 훈련된 모델을 훈련하는 것이 포함됩니다. ChatGPT를 예로 들어보겠습니다.
GPT 모델을 사용해 본 적이 있다면 "이것을 작성하라"는 메시지보다는 다음과 같은 메시지가 더 많다는 것을 알고 계실 것입니다.
- 프롬프트: 옛날 옛적에
- 계속: 탑 꼭대기에는 사악한 마법사가 있었습니다.
- 계속: 탑 꼭대기에는 사악한 마법사가 있었습니다.
- 프롬프트 : 치킨은 왜 밴드에 가입했나요?
- 계속 : 드럼스틱이 있었기 때문이죠!
그 시점에서 ChatGPT에 도달하려면 많은 저임금 노동이 필요합니다. 그 사람들은 GPT 응답과 예상되는 행동의 중요성을 파악하기 위해 엄청난 양의 말뭉치를 만듭니다. 이 작업자는 다음과 같은 수많은 프롬프트/계속 텍스트를 생성합니다.
- 프롬프트 : 이 이야기를 마무리하세요: "옛날 옛적에.."
- 계속 : 물론이죠! 옛날, 머나먼 땅에 장엄한 두 산 사이에 자리잡은 작은 마을이 있었습니다.
- 계속 : 물론이죠! 옛날, 머나먼 땅에 장엄한 두 산 사이에 자리잡은 작은 마을이 있었습니다.
- 프롬프트 : 닭에 관한 농담을 들려주세요.
- 계속 : 치킨은 왜 밴드에 가입했나요? 왜냐면 드럼스틱이 있었거든요!
이러한 미세 조정 프로세스는 다음과 같은 여러 가지 이유로 필수적입니다.
- 특이성: 사전 훈련을 통해 모델은 언어에 대한 폭넓은 이해를 얻을 수 있지만, 미세 조정을 통해 지식과 행동의 범위를 좁혀 특정 작업이나 영역에 더 부합하게 됩니다. 예를 들어, 의료 데이터에 대해 미세 조정된 모델은 의학적 질문에 더 나은 답변을 제공합니다.
- 제어: 미세 조정을 통해 개발자는 모델의 출력을 더 효과적으로 제어할 수 있습니다. 개발자는 선별된 데이터 세트를 사용하여 모델이 원하는 응답을 생성하고 원치 않는 동작을 방지하도록 안내할 수 있습니다.
- 안전: 유해하거나 편향된 출력을 줄이는 데 도움이 됩니다. 검토자는 세부 조정 과정에서 지침을 사용하여 모델이 부적절한 콘텐츠를 생성하지 않도록 할 수 있습니다.
- 성능: 미세 조정을 통해 특정 작업에 대한 모델 성능을 크게 향상시킬 수 있습니다. 예를 들어, 고객 지원을 위해 미세 조정된 모델은 일반 모델보다 훨씬 더 나은 성능을 발휘합니다.
ChatGPT가 특히 어떤 면에서 미세 조정되었음을 알 수 있습니다.
예를 들어, "논리적 추론"은 LLM이 어려움을 겪는 경향이 있습니다. ChatGPT의 최고의 논리적 추론 모델인 GPT-4는 숫자의 패턴을 명시적으로 인식하도록 집중적으로 훈련되었습니다.
다음과 같은 것 대신에:
- 프롬프트 : 2+2가 무엇인가요?
- 과정 : 2+2=4 어린이를 위한 수학 교과서에 자주 등장합니다. 때때로 "2+2=5"에 대한 언급이 있지만, 그런 경우에는 일반적으로 George Orwell이나 Star Trek과 관련된 더 많은 맥락이 있습니다. 이것이 그러한 맥락에서라면 가중치는 2+2=5에 더 유리할 것입니다. 그러나 해당 컨텍스트는 존재하지 않으므로 이 경우 다음 토큰은 4일 가능성이 높습니다.
- 응답 : 2+2=4
훈련은 다음과 같이 수행됩니다.
- 훈련: 2+2=4
- 훈련: 4/2=2
- 훈련: 4의 절반은 2
- 훈련: 2/2는 4
…등등.
이는 보다 "논리적인" 모델의 경우 훈련 프로세스가 더욱 엄격해지고 모델이 논리적, 수학적 원리를 이해하고 올바르게 적용하도록 하는 데 중점을 둡니다.
모델은 다양한 수학적 문제와 그 솔루션에 노출되어 이러한 원칙을 새로운, 보이지 않는 문제에 일반화하고 적용할 수 있도록 보장합니다.
특히 논리적 추론을 위한 이러한 미세 조정 프로세스의 중요성은 아무리 강조해도 지나치지 않습니다. 이것이 없으면 모델은 간단한 논리적 또는 수학적 질문에 대해 부정확하거나 무의미한 답변을 제공할 수 있습니다.
이미지 모델과 언어 모델
이미지 모델과 언어 모델 모두 변환기와 같은 유사한 아키텍처를 사용할 수 있지만 처리하는 데이터는 근본적으로 다릅니다.
이미지 모델
이러한 모델은 픽셀을 처리하고 종종 계층적 방식으로 작동하여 작은 패턴(예: 가장자리)을 먼저 분석한 다음 이를 결합하여 더 큰 구조(예: 모양)를 인식하는 등 전체 이미지를 이해할 때까지 계속됩니다.
언어 모델
이러한 모델은 단어나 문자의 시퀀스를 처리합니다. 일관되고 문맥상 관련성이 높은 텍스트를 생성하려면 문맥, 문법, 의미론을 이해해야 합니다.
탁월한 생성 AI 인터페이스의 작동 방식
Dall-E + 미드저니
Dall-E는 이미지 생성에 적합한 GPT-3 모델의 변형입니다. 텍스트-이미지 쌍으로 구성된 방대한 데이터 세트에 대해 훈련되었습니다. Midjourney는 독점 모델을 기반으로 하는 또 다른 이미지 생성 소프트웨어입니다.
- 입력: "머리가 둘 달린 플라밍고"와 같은 텍스트 설명을 제공합니다.
- 처리: 이러한 모델은 이 텍스트를 일련의 숫자로 인코딩한 다음 이러한 벡터를 디코딩하여 픽셀과의 관계를 찾아 이미지를 생성합니다. 모델은 훈련 데이터로부터 텍스트 설명과 시각적 표현 사이의 관계를 학습했습니다.
- 출력: 주어진 설명과 일치하거나 관련된 이미지입니다.
손가락, 패턴, 문제
이러한 도구가 정상적으로 보이는 손을 지속적으로 생성할 수 없는 이유는 무엇입니까? 이러한 도구는 서로 옆에 있는 픽셀을 확인하여 작동합니다.
이전 또는 더 원시적으로 생성된 이미지를 최신 이미지와 비교할 때 이것이 어떻게 작동하는지 확인할 수 있습니다. 이전 모델은 매우 흐릿해 보입니다. 대조적으로, 최신 모델은 훨씬 더 선명합니다.
이러한 모델은 이미 생성된 픽셀을 기반으로 다음 픽셀을 예측하여 이미지를 생성합니다. 이 과정은 완전한 이미지를 생성하기 위해 수백만 번 이상 반복됩니다.
손, 특히 손가락은 복잡하고 정확하게 캡처해야 할 세부 사항이 많습니다.
각 손가락의 위치, 길이 및 방향은 이미지에 따라 크게 다를 수 있습니다.
텍스트 설명에서 이미지를 생성할 때 모델은 손의 정확한 자세와 구조에 대해 많은 가정을 해야 하며, 이로 인해 이상이 발생할 수 있습니다.
채팅GPT
ChatGPT는 자연어 처리 작업을 위해 설계된 변환기 기반 모델인 GPT-3.5 아키텍처를 기반으로 합니다.
- 입력: 대화를 시뮬레이션하기 위한 프롬프트 또는 일련의 메시지입니다.
- 처리: ChatGPT는 다양한 인터넷 텍스트의 방대한 지식을 사용하여 응답을 생성합니다. 대화에 제공된 맥락을 고려하여 가장 관련성이 높고 일관성 있는 답변을 생성하려고 노력합니다.
- 출력: 대화를 계속하거나 응답하는 텍스트 응답입니다.
전문
ChatGPT의 강점은 다양한 주제를 처리하고 인간과 같은 대화를 시뮬레이션하는 능력에 있어 챗봇과 가상 비서에 이상적입니다.
Bard + 검색 생성 경험(SGE)
특정 세부 사항은 독점적일 수 있지만 Bard는 다른 최첨단 언어 모델과 유사한 변환기 AI 기술을 기반으로 합니다. SGE는 유사한 모델을 기반으로 하지만 Google이 사용하는 다른 ML 알고리즘을 사용합니다.
SGE는 변환기 기반 생성 모델을 사용하여 콘텐츠를 생성한 다음 퍼지가 검색 순위 페이지에서 답변을 추출합니다. (이것은 사실이 아닐 수도 있습니다. 그냥 가지고 놀면서 어떻게 작동하는지에 근거한 추측일 뿐입니다. 저를 고소하지 마세요!)
- 입력: 프롬프트/명령/검색
- 처리: Bard는 입력을 처리하고 다른 LLM과 같은 방식으로 작동합니다. SGE는 유사한 아키텍처를 사용하지만 적절한 응답을 생성하기 위해 내부 지식(훈련 데이터에서 얻은)을 검색하는 레이어를 추가합니다. 프롬프트의 구조, 맥락, 의도를 고려하여 관련 콘텐츠를 생성합니다.
- 출력: 스토리, 답변 또는 기타 유형의 텍스트일 수 있는 생성된 콘텐츠입니다.
생성적 AI의 적용(및 그에 대한 논란)
예술과 디자인
이제 제너레이티브 AI(Generative AI)는 예술 작품, 음악, 심지어 제품 디자인까지 만들 수 있습니다. 이는 창의성과 혁신을 위한 새로운 길을 열었습니다.
논쟁
예술 분야에서 AI의 등장은 창작 분야에서 일자리 감소에 대한 논쟁을 촉발시켰습니다.
추가적으로 다음과 같은 우려사항이 있습니다.
- 노동 위반, 특히 AI 생성 콘텐츠가 적절한 귀속이나 보상 없이 사용되는 경우.
- 작가들을 AI로 대체하겠다고 경영진이 위협하는 것도 작가 파업을 촉발한 이슈 중 하나다.
자연어 처리(NLP)
AI 모델은 이제 챗봇, 언어 번역 및 기타 NLP 작업에 널리 사용됩니다.
일반 인공 지능(AGI)의 꿈을 제외하면 LLM은 "일반주의" NLP 모델에 가깝기 때문에 LLM을 가장 잘 활용하는 방법입니다.
논쟁
많은 사용자는 챗봇이 비개인적이고 때로는 짜증스럽다고 생각합니다.
더욱이 AI는 언어 번역 분야에서 상당한 진전을 이루었지만 인간 번역가가 가져오는 뉘앙스와 문화적 이해가 부족하여 인상적이고 결함이 있는 번역으로 이어지는 경우가 많습니다.
의학 및 약물 발견
AI는 방대한 양의 의료 데이터를 신속하게 분석하고 잠재적인 약물 화합물을 생성하여 약물 발견 프로세스를 가속화할 수 있습니다. 많은 의사들이 이미 LLM을 사용하여 메모와 환자 커뮤니케이션을 작성하고 있습니다.
논쟁
의료 목적으로 LLM에 의존하는 것은 문제가 될 수 있습니다. 의학에는 정확성이 필요하며 AI의 실수나 실수는 심각한 결과를 초래할 수 있습니다.
의학에는 이미 LLM을 사용할 때 더 많이 구워지는 편견이 있습니다. 아래에 설명된 것처럼 개인 정보 보호, 효율성 및 윤리와 관련된 유사한 문제도 있습니다.
노름
많은 AI 애호가들은 AI를 게임에 활용하는 것에 열광하고 있습니다. 그들은 AI가 현실적인 게임 환경, 캐릭터, 심지어 전체 게임 플롯까지 생성하여 게임 경험을 향상시킬 수 있다고 말합니다. NPC 대화는 이러한 도구를 사용하여 향상될 수 있습니다.
논쟁
게임 디자인의 의도성에 대한 논쟁이 있습니다.
AI는 방대한 양의 콘텐츠를 생성할 수 있지만 일부에서는 인간 디자이너가 가져오는 의도적인 디자인과 내러티브 응집력이 부족하다고 주장합니다.
Watchdogs 2에는 프로그래밍 방식의 NPC가 있었는데, 이는 게임 전체의 내러티브 응집력을 거의 추가하지 못했습니다.
마케팅과 광고
AI는 소비자 행동을 분석하고 개인화된 광고 및 홍보 콘텐츠를 생성하여 마케팅 캠페인을 더욱 효과적으로 만들 수 있습니다.
LLM은 다른 사람의 글에서 얻은 맥락을 갖고 있으므로 사용자 스토리나 보다 미묘한 프로그래밍 아이디어를 생성하는 데 유용합니다. 방금 TV를 구입한 사람에게 TV를 추천하는 대신 LLM은 누군가가 원하는 액세서리를 추천할 수 있습니다.
논쟁
마케팅에 AI를 사용하면 개인정보 보호에 대한 우려가 높아집니다. 소비자 행동에 영향을 미치기 위해 AI를 사용하는 것의 윤리적 의미에 대한 논쟁도 있습니다.
더 자세히 알아보기: 마케팅에서 대규모 언어 모델의 사용을 확장하는 방법
Continuing issues with LLMS
Contextual understanding and comprehension of human speech
- Limitation: AI models, including GPT, often struggle with nuanced human interactions, such as detecting sarcasm, humor, or lies.
- Example: In stories where a character is lying to other characters, the AI might not always grasp the underlying deceit and might interpret statements at face value.
Pattern matching
- Limitation: AI models, especially those like GPT, are fundamentally pattern matchers. They excel at recognizing and generating content based on patterns they've seen in their training data. However, their performance can degrade when faced with novel situations or deviations from established patterns.
- Example: If a new slang term or cultural reference emerges after the model's last training update, it might not recognize or understand it.
Lack of common sense understanding
- Limitation: While AI models can store vast amounts of information, they often lack a "common sense" understanding of the world, leading to outputs that might be technically correct but contextually nonsensical.
Potential to reinforce biases
- Ethical consideration: AI models learn from data, and if that data contains biases, the model will likely reproduce and even amplify those biases. This can lead to outputs that are sexist, racist, or otherwise prejudiced.
Challenges in generating unique ideas
- Limitation: AI models generate content based on patterns they've seen. While they can combine these patterns in novel ways, they don't "invent" like humans do. Their "creativity" is a recombination of existing ideas.
Data Privacy, Intellectual Property, and Quality Control Issues:
- Ethical consideration : Using AI models in applications that handle sensitive data raises concerns about data privacy. When AI generates content, questions arise about who owns the intellectual property rights. Ensuring the quality and accuracy of AI-generated content is also a significant challenge.
Bad code
- AI models might generate syntactically correct code when used for coding tasks but functionally flawed or insecure. I have had to correct the code people have added to sites they generated using LLMs. It looked right, but was not. Even when it does work, LLMs have out-of-date expectations for code, using functions like “document.write” that are no longer considered best practice.
Hot takes from an MLOps engineer and technical SEO
This section covers some hot takes I have about LLMs and generative AI. Feel free to fight with me.
Prompt engineering isn't real (for generative text interfaces)
Generative models, especially large language models (LLMs) like GPT-3 and its successors, have been touted for their ability to generate coherent and contextually relevant text based on prompts.
Because of this, and since these models have become the new “gold rush," people have started to monetize “prompt engineering” as a skill. This can be either $1,400 courses or prompt engineering jobs.
However, there are some critical considerations:
LLMs change rapidly
As technology evolves and new model versions are released, how they respond to prompts can change. What worked for GPT-3 might not work the same way for GPT-4 or even a newer version of GPT-3.
This constant evolution means prompt engineering can become a moving target, making it challenging to maintain consistency. Prompts that work in January may not work in March.
Uncontrollable outcomes
While you can guide LLMs with prompts, there's no guarantee they'll always produce the desired output. For instance, asking an LLM to generate a 500-word essay might result in outputs of varying lengths because LLMs don't know what numbers are.
Similarly, while you can ask for factual information, the model might produce inaccuracies because it cannot tell the difference between accurate and inaccurate information by itself.
Using LLMs in non-language-based applications is a bad idea
LLMs are primarily designed for language tasks. While they can be adapted for other purposes, there are inherent limitations:
Struggle with novel ideas
LLMs are trained on existing data, which means they're essentially regurgitating and recombining what they've seen before. They don't "invent" in the truest sense of the word.
Tasks that require genuine innovation or out-of-the-box thinking should not use LLMs.
You can see an issue with this when it comes to people using GPT models for news content – if something novel comes along, it's hard for LLMs to deal with it.
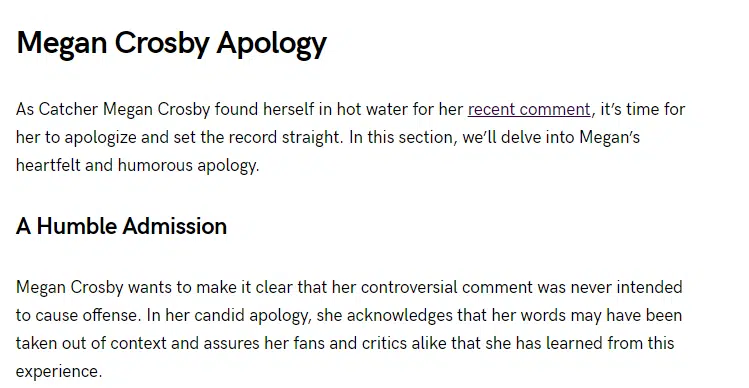
For example, a site that seems to be generating content with LLMs published a possibly libelous article about Megan Crosby. Crosby was caught elbowing opponents in real life.
Without that context, the LLM created a completely different, evidence-free story about a “controversial comment.”
Text-focused
At their core, LLMs are designed for text. While they can be adapted for tasks like image generation or music composition, they might not be as proficient as models specifically designed for those tasks.
LLMs don't know what the truth is
They generate outputs based on patterns encountered in their training data. This means they can't verify facts or discern true and false information.
If they've been exposed to misinformation or biased data during training, or they don't have context for something, they might propagate those inaccuracies in their outputs.
This is especially problematic in applications like news generation or academic research, where accuracy and truth are paramount.
Think about it like this: if an LLM has never come across the name “Jimmy Scrambles” before but knows it's a name, prompts to write about it will only come up with related vectors.
Designers are always better than AI-generated Art
AI has made significant strides in art, from generating paintings to composing music. However, there's a fundamental difference between human-made art and AI-generated art:
Intent, feeling, vibe
Art is not just about the final product but the intent and emotion behind it.
A human artist brings their experiences, emotions, and perspectives to their work, giving it depth and nuance that's challenging for AI to replicate.
A “bad” piece of art from a person has more depth than a beautiful piece of art from a prompt.
이 기사에 표현된 의견은 게스트 작성자의 의견이며 반드시 Search Engine Land일 필요는 없습니다. 직원 작성자는 여기에 나열되어 있습니다.
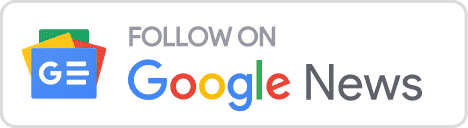